When you go to a new healthcare clinic in the United States, doctors and nurses pull up your patient record based on your name and birthdate. Sometimes it’s not your chart they pull up. This is not only a healthcare problem; it’s a data science problem.
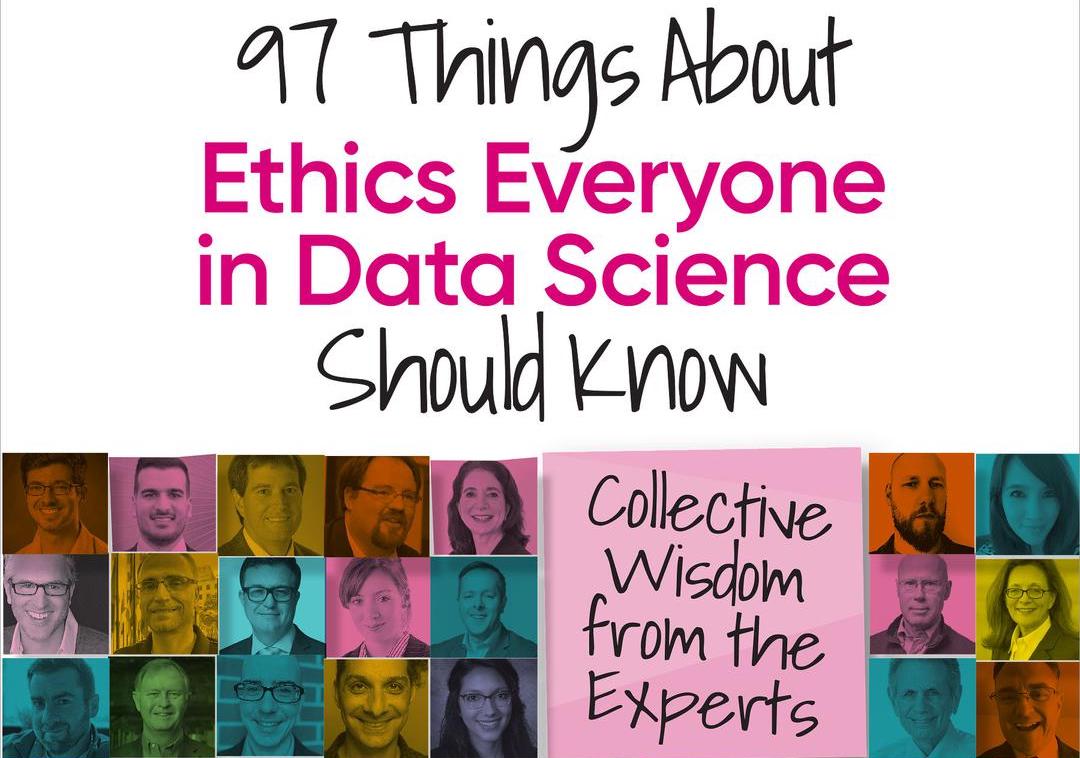
This article appeared in the O’Reilly Media book 97 Things about Ethics Everyone in Data Science Should Know.
Two things (at least) contribute to error: a lack of consistent and uniform patient records, and secondly, public mistrust in protection of data. Both of them hold back healthcare from data science revolutions.
In transferring patient records from one major hospital system to another, patient data passes through health information exchanges. The current rate of correctly matching patients between systems is estimated to be around 30%.1 With considerable effort from data scientists into data cleaning and better algorithms, we could potentially match as often as 95%. This is an important opportunity for data science to improve healthcare! It’s called “master data management” or “data governance” and, while we have a long way to go, we’re getting better.
The healthcare industry works hard to prevent misidentification. Using at least two patient identifiers, such as name and birthdate, is standard practice.2 Unfortunately, name and birthdate do not uniquely identify a patient. A third identifier should also be used. There are many choices: hospital ID, Social Security number, a wristband with barcodes, photographs, and two-factor authentication devices. A third identifier, or even a fourth, won’t solve the problem. Humans performing repetitive processes, even under ideal circumstances, are only accurate 99.98% of the time. In high-stress situations like medical emergencies, accuracy rates fall to about 92%.3
Computers supplement healthcare workers’ accuracy. Most of the United States healthcare system uses statistical matching of multiple patient attributes.4 An alert notifies users that a patient is statistically similar to another patient. Even after decades of improvement, however, medical errors persist.
While excellent master data management can bring us to 95% correct identification rate in health information exchanges, some have concluded the only way to improve to 99% is by adopting a universal patient ID.5 Simply put, if society decides to prioritize patient identification, it must be willing to accept a universal patient ID. Master data management, corporate consolidation, Social Security numbers, and national health coverage are all consistent with the use of universal patient IDs.
A universal patient ID may seem inevitable, but it is not. Many organizations have good cause to resist a universal ID or database. As data scientists we appreciate the American Civil Liberties Union’s argument that any nationwide ID will lead to surveillance and monitoring of citizens.6 The ECRI Institute, a healthcare research organization, identifies understandable cultural and social barriers to patient ID policies.7 The National Rifle Association has successfully resisted a searchable database of gun owners.8
This is where we come in. Before society readily accepts a universal ID, the data science field must demonstrate that users’ privacy can and will be maintained. Our challenge is to ensure that people have autonomy over how their data can be used and who can use it. We must prevent catastrophic data breaches like Equifax, or unethical data mining from the likes of Cambridge Analytica and Facebook and Target.9,10,11 We must build something we have not yet earned: trust.
Securing private data against breaches is hard, costly, and takes vigilance. The ethical treatment of data also comes at a cost—exploitation is often profitable! A universal ID would be a powerful, exploitable tool that invites data breaches. We are not ready for it, but we could be, once we build the public’s trust.
Building trust and appropriate data governance—this is how we eliminate medical error.
Sources
[1] RAND Corporation, “Defining and Evaluating Patient-Empowered Approaches to Improving Record Matching”, (2018), https://rand.org/t/RR2275
[2] World Health Organization, “Patient Identification”, (2007), https://www.who.int/patientsafety/solutions/patientsafety/PS-Solution2.pdf
[3] Fred Trotter and David Uhlman, Hacking Healthcare, O’Reilly Media, (2011), https://shop.oreilly.com/product/0636920020110.do
[4] RAND Corporation, “Identity Crisis: An Examination of the Costs and Benefits of a Unique Patient Identifier for the U.S. Health Care System”, (2008), https://rand.org/t/MG753
[5] ECRI Institute, “Patient Identification Errors”, (2016), https://ecri.org/Resources/HIT/Patient%20ID/Patient_Identification_Evidence_Based_Literature_final.pdf
[6] American Civil Liberties Union, “5 Problems with National ID Cards”, https://www.aclu.org/other/5-problems-national-id-cards
[7] ECRI Institute “ECRI PSO Deep Dive: Patient Identification”, (2016), https://ecri.org/patient-identification-deep-dive
[8] Jeanne Marie Laskas “Inside the Federal Bureau Of Way Too Many Guns”, GQ, (2016), https://www.gq.com/story/inside-federal-bureau-of-way-too-many-guns
[9] Federal Trade Commission, “Equifax Data Breach Settlement”, (2020), https://ftc.gov/enforcement/cases-proceedings/refunds/equifax-data-breach-settlement
[10] Wikipedia contributors, “Facebook–Cambridge Analytica data scandal”, Wikipedia, The Free Encyclopedia, (2020), https://en.wikipedia.org/wiki/Facebook-Cambridge_Analytica_data_scandal
[11] Charles Duhigg, “How Companies Learn Your Secrets”, The New York Times Magazine, (2012), https://nytimes.com/2012/02/19/magazine/shopping-habits.html